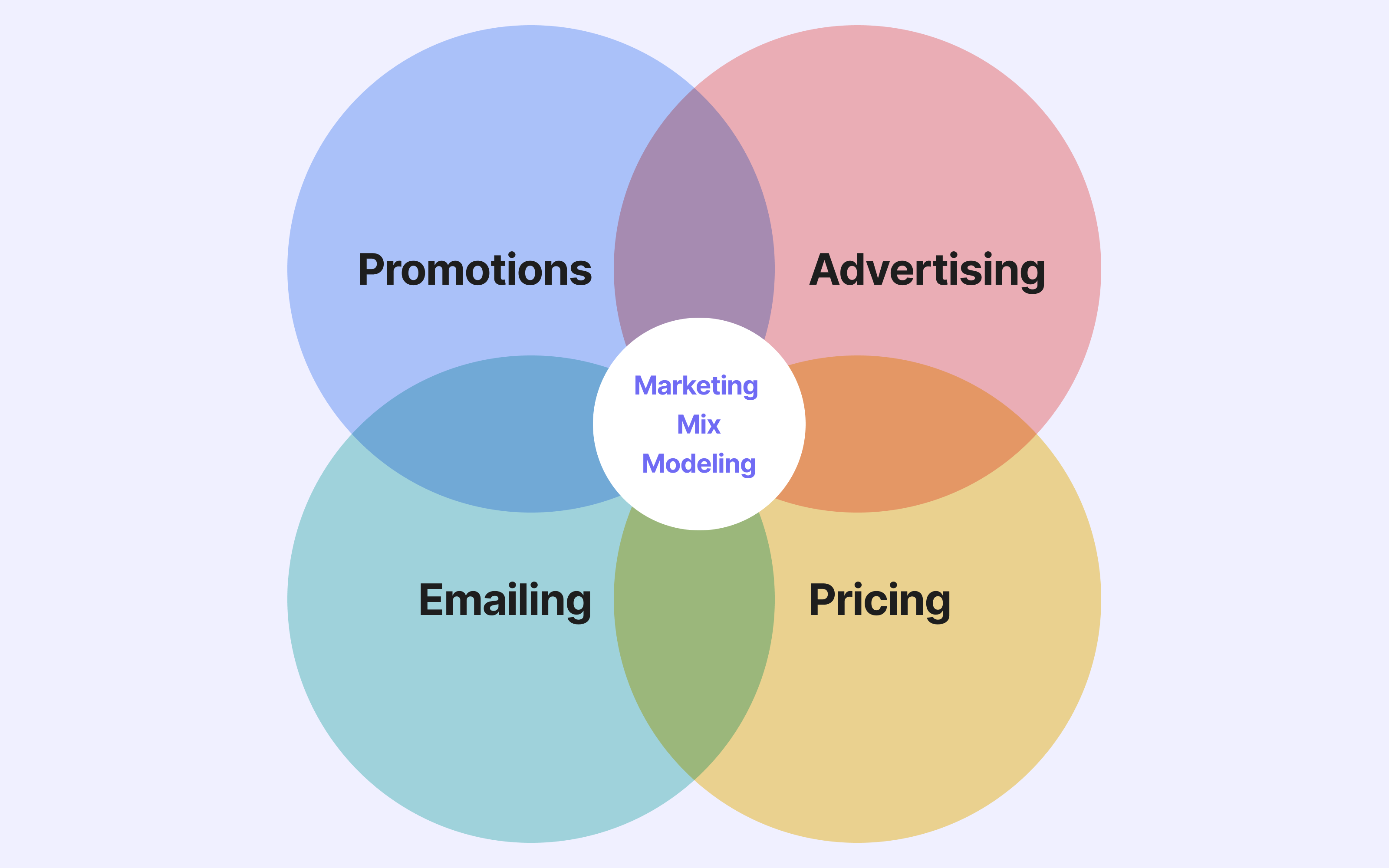
Marketing Mix Modeling: Measure Marketing impacts
7min • Last updated on May 7, 2025
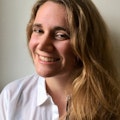
Alexandra Augusti
Chief of Staff
Measuring marketing performance with MMM
Every marketing action has an impact, but how can you measure it accurately in a world dominated by data? You have certainly wondered how to measure the impact of your marketing investments on performance and sales. To accurately assess these performances, it is essential to use quantitative measures that reflect the outcomes achieved, both in terms of sales and visibility.
With the gradual disappearance of third-party cookies, Marketing Mix Modeling (MMM) is now widely endorsed because it does not rely on individual tracking.
MMM can be more or less complex, based on multi-linear regression techniques or Bayesian models.
💡 Don't worry, this article aims to explain what it is concretely and how to implement an MMM project.
[Updated on 5 February 2025]
Google has just made Meridian*, its open-source MMM tool, available to the public. This solution helps advertisers optimise their budgets by identifying the most effective channels.
What is Marketing Mix Modeling?
Definition
First thing first: what is Marketing Mix Modeling? 🤔
Marketing Mix Modeling is a quantitative technique used to understand the impact of various marketing actions on overall performance, and thus measure ROI.
MMM analyzes historical data to identify the relationships between the different components of the mix (advertising, promotions, pricing, channels, content etc.) and business outcomes.
It enables businesses to assess the actual impact of each marketing channel—whether paid, owned, or earned (POE)—on overall performance. By analysing advertising spend and sales over multiple years, they can identify patterns and adjust their strategies accordingly.
This in-depth analysis helps the company gather valuable insights for better decision-making, particularly in choosing the independent variables involved in MMM (see the following section).
This model is widely used in various sectors, from consumer goods to financial services, to efficiently allocate media and marketing investments. According to a study conducted in 2024, more than one in two American marketers already use a Marketing Mix Modeling approach (eMarketer).
In summary, Marketing Mix Modeling is essential for optimizing resource allocation (budgetary, or even human) across different media and channels, as well as for defining new marketing strategies and predicting future performance.
Comparison with attribution models
Be careful not to confuse MMM with techniques such as marketing attribution, which focuses on evaluating individual digital channels rather than the entire mix. MMM and traditional attribution models differ in several ways:
MMM is based on historical data, while marketing attribution analyzes behavioral data almost in real time.
MMM focuses on the entire mix and includes non-digital actions like traditional media, billboards, etc.
MMM uses more complex mathematical techniques (regression, Bayesian models, etc.) while marketing attribution relies on predefined models to determine the acquisition channel that led to the conversion (last click, first touch, etc.).
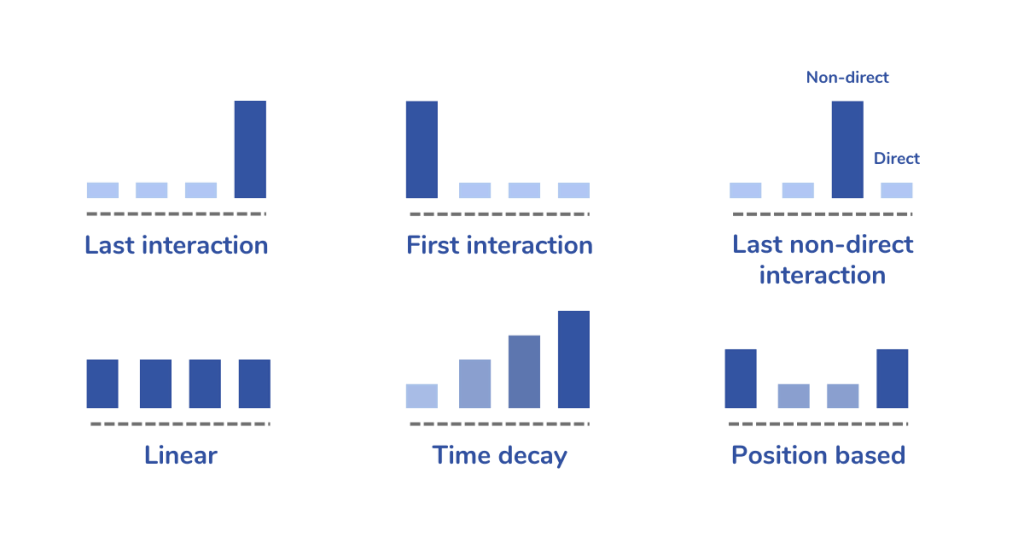
Examples of marketing attribution models
MMM does not rely on individual tracking of consumers, which is beneficial with the end of third-party cookies.
By implementing strategies based on MMM, a company can not only measure the impact of each lever but also significantly improve its overall marketing performance.
How to Implement Your Marketing Mix Modeling?
Starting an MMM project requires a deep understanding of the company and its long-term objectives. Here are the steps to follow to successfully carry out your Marketing Mix Modeling project.
Step 1: Data Collection and Preparation
Data quality is the cornerstone of effective MMM. Indeed, collecting data from various sources—sales, media, demographics—ensures a comprehensive understanding of the factors influencing performance.
We recommend using a cloud data warehouse to centralize these data for subsequent analysis. First-party data collection is the foundation of any cookieless solution.
Additionally, data preparation is also critical. This includes data cleaning (eliminating errors, managing missing values) and structuring for analysis. Underestimating the importance of this step can compromise the quality of the final model and its conclusions.
Step 2: Choosing Variables
To build a robust MMM model, it is essential to choose the right variables:
The dependent variable, which corresponds to the performance you want to optimize (most often, sales).
The independent variables, which influence the dependent variable. These generally correspond to different marketing actions and external elements (market trends, seasonality, etc.).
The relevance of the explanatory variables chosen for the model must be validated using statistical techniques to ensure that they are significantly related to the measured outcomes. For example, using correlation analysis can reveal the importance of certain advertising expenditures on sales.
Neglecting interactions between variables, such as the combined effect of TV advertising and in-store promotions, can skew the results.
Step 3: Constructing the MMM
Constructing the model is obviously the key implementation step of a Marketing Mix Modeling method. Two main methods are used to construct the MMM:
Multi-linear regression allows analyzing the combined effect of several independent variables on a dependent variable (i.e., sales in most cases).
This method provides a precise understanding of the individual contribution of each factor in the overall results, thereby facilitating a more strategic and informed resource allocation.
Bayesian modeling offers the flexibility to incorporate prior knowledge and specific assumptions into the predictive analysis. Organizations can thus customize the model's predictions to better reflect specific insights or integrate information that is hard to quantify.
This approach is particularly advantageous in scenarios where empirical data are incomplete or uncertain, allowing for more robust decision-making based on expert knowledge and adjusted forecasts.
Note, this step requires skills in statistics, programming, and marketing if you want to develop your own Marketing Mix Modeling in-house.
Otherwise, you can use:
Open-source models, like Meridian developed by Google or Robyn, offered by Meta.
Paid platforms specializing in MMM, like Rockerbox or Recast.
Step 4: Validating Results
After constructing the models, it is essential to validate them to ensure their reliability.
Using advanced estimation methods ensures the reliability of the results obtained. Interpreting the coefficients, which quantify the impact of each mix variable on sales, must be done cautiously, taking into account the business objectives of the company.
It is critical not to over-interpret coefficients that are not statistically significant, as this could lead to incorrect marketing decisions.
🛠 If necessary, you can iterate steps 2, 3, and 4 until you are fully satisfied.
Step 5: Using Results to Optimize Marketing Strategies
The insights generated by the MMM must be actively used to refine marketing strategies. An analysis phase is essential to understand the effectiveness of each marketing lever/action.
The model's results can guide decisions on the allocation of marketing budgets. Practical cases show how adjustments based on MMM results have significantly improved marketing performance.
By taking the time to dissect the results, you can understand which media is most effective, which advertising needs to be optimized, and which investments need to be rethought to ensure a better ROI from your marketing strategy.
⚠️ Be sure to test the recommendations on a limited market segment before a large-scale deployment to validate the model's assumptions.
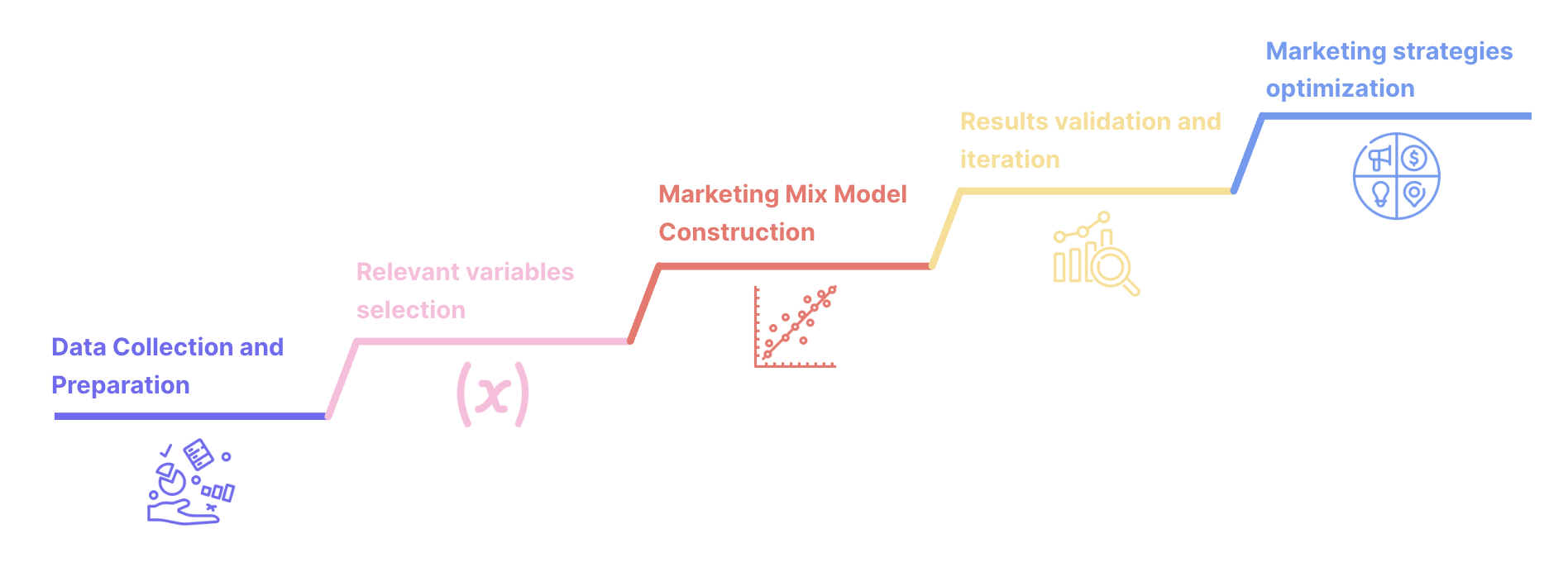
Marketing Mix Modeling: detailed steps
Limitations and Challenges of Marketing Mix Modeling
Like any methodology, MMM is not perfect, and understanding its limitations is essential for using it intelligently:
MMM can be limited by external factors which may not be measured, such as changes in competition or the economy. Integrating real-time data can help overcome some of these challenges by making the model more responsive to current market conditions.
The quality and quantity of historical data strongly determine the accuracy of the model. Without sufficient history, MMM will not be relevant. Moreover, historical performance does not always guarantee similar future results. Therefore, it is important to apply a cautious approach using the results of the MMM as a guide rather than a path to follow blindly.
👉🏼 Do not hesitate to consider market conditions, brand sentiment, and competitive campaigns in your decisions.
Another challenge is accessing data outside the digital environment: TV, print media, billboards, etc. Some advertisers do not have the capability to centralize data from all marketing channels. To address this challenge, we always recommend centralizing 100% of your data in a data warehouse.
Conclusion
Marketing Mix Modeling is a powerful tool for businesses looking to optimize their marketing impact through rigorous data and statistical analyses. Don't hesitate any longer and incorporate MMM into your marketing strategy to strengthen your decisions based on tangible evidence and effectively manage your marketing investments.
About DinMo: DinMo is at the forefront of the next generation of Customer Data Platforms with our composable CDP. We help our customers transform their data into profitable growth and measure their marketing impact. For more information about DinMo, feel free to contact us!
*Source: Google